Artificial Intelligence and a Low-cost EEG to Identify Mental Health Disorders
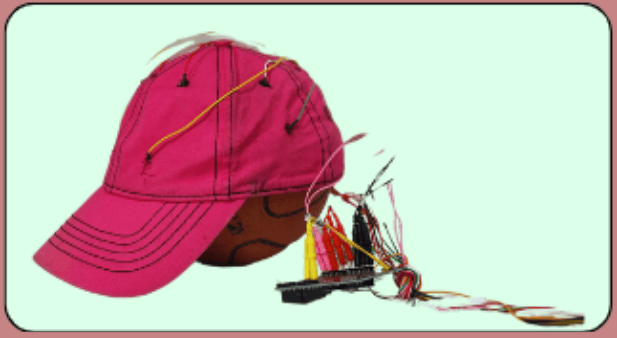
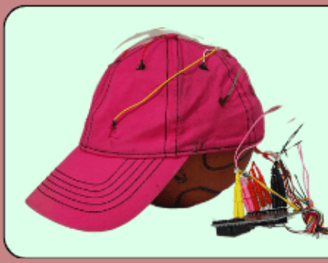
Utilizing artificial intelligence (AI) in conjunction with affordable electroencephalography (EEG) technology holds promise for identifying and understanding mental health disorders more effectively and economically. By harnessing AI algorithms trained on EEG data, researchers and clinicians can potentially detect patterns or biomarkers associated with various mental health conditions such as depression, anxiety, and schizophrenia. These AI-driven analyses may offer insights into underlying neurological mechanisms and aid in early diagnosis and personalized treatment planning.
The integration of low-cost EEG devices makes this approach more accessible and scalable, particularly in resource-limited settings or for remote monitoring. These devices, often wearable and non-invasive, enable continuous data collection outside clinical settings, providing a more comprehensive picture of an individual's brain activity in real-world contexts.
“In countries of all income levels, people who are depressed are often not correctly diagnosed, and others who do not have the disorder are too often misdiagnosed and prescribed antidepressants,” WHO warns
Bhavna is a low-cost electroencephalogram that uses cheap electronics such as an arduino board and electrodes and an Artificial Intelligence model to identify 16 of the most common disorders in the world.
According to the World Health Organization (WHO), 970 million people suffer from a mental health disorder globally. Our current mental health diagnostic process is expensive, long, and inaccurate. According to Substance Abuse and Mental Helath Services Administration (SAMHSA), cost is the top factor why people don’t look for mental health services. The diagnostic process has 3 steps- the psychological evaluation, the physical exam, and the lab test. This procedure takes at least 3 weeks’ time for the result. As the patients wait for the result, the disorder is gradually worsening and making it harder to cure. On an average, the accuracy rate of a mental health disorder is less than 50%, starting as low as 0%.
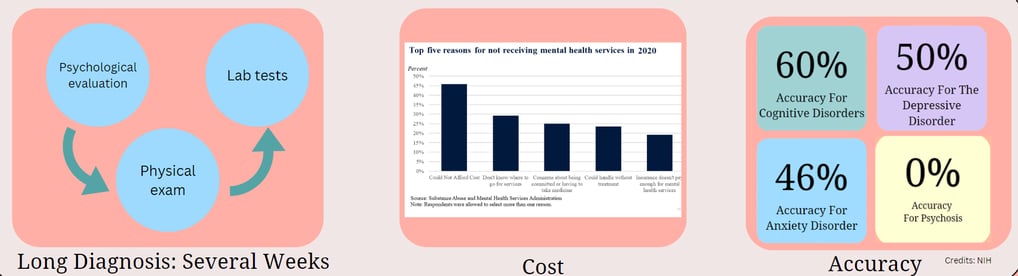
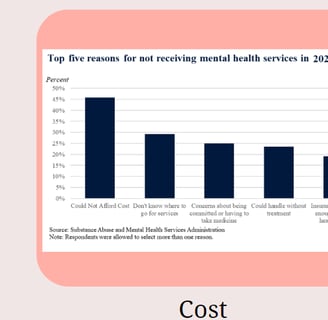
Problem with Current Diagnostic Process
Solution

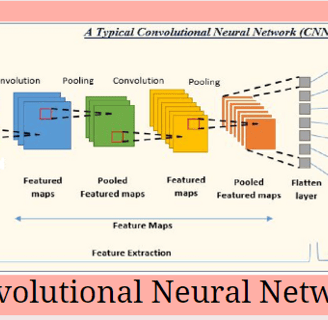
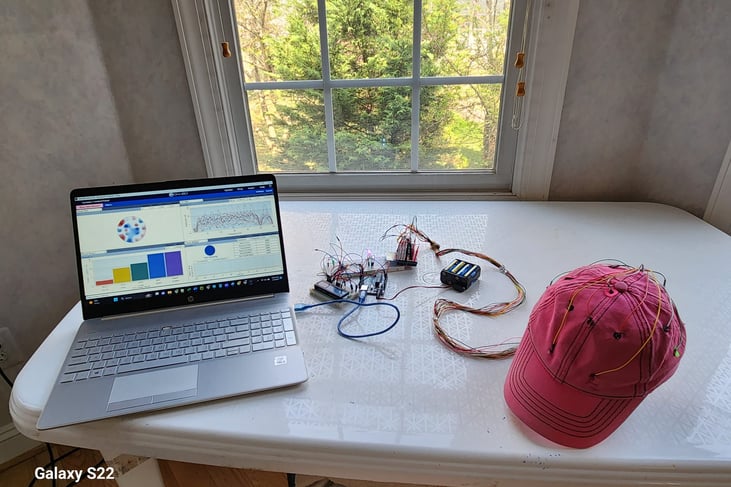
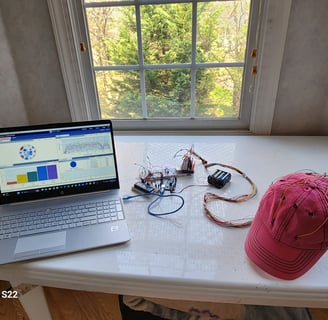
There are 2 main components of my device. One is the physical EEG and the second is the Machine Learning model. The EEG cap detects the brain waves based off of the signals that the electrodes recieve. Next, these signals are inputed into the Machine Learning model for the software to compare the raw EEG data to the dataset and to eventually determine and identify the mental health disorder(s). The EEG cap is made of cheap electronics such as electrodes and an arduino as an amplifier to move the data to the Elastic Net algorithm. The dataset has over 1,800,000 records of data.
Bhavna Foundation
info@bhavnafoundation.org
(703)-869-0374